Self hosted tools Benchmarking
- Aman Gupta,
Jr. Data Engineer
Almost every data stack has SQL as a source. Since SQL RDBMS hasn't really changed how they work fundamentally over the years, you'd expect SQL to SQL copy to be a long solved problem, but as we can see, it's still a challenge people are solving in 2024.
Our benchmark approach
Let’s benchmark the existing OSS solutions for this. Here are the tools we tested:
- Meltano - v3.5.4
- Airbyte - v1.1.0
- dlt - v1.2.0
- Sling - 1.2.2
We used the latest versions of the tools available at the time of testing.
The dataset: TPC-H benchmark
We used the industry-standard TPC-H dataset for our benchmark, consisting of:
- Total size: ~6.42 GB in PostgreSQL
- 7 tables
- 24,604,860 rows
Environment Used
Hosted on Google Cloud VM with the following setup:
- CPUs: 8 cores
- Memory: 16 GB RAM
- CPU Base Frequency: 2.3 GHz
Setup
Each tool's configuration is closely aligned with typical deployment practices. Here’s a brief description of setting up each tool.
1. dlt
- Installed via
pip
and initialized withdlt init
. - Configured with
config.toml
andsecrets.toml.
- The pipeline was executed using
sql_database_pipeline.py
.
2. Airbyte
- Runs locally with
abctl
on the VM. - The setup involves configuring sources and destinations through Airbyte’s web UI for interactive pipeline management.
3. Meltano
- Installed on the VM and configured for running taps and loaders.
meltano.yml.
- It includes setup for
tap-postgres
andtarget-bigquery
, with batch processing in JSONL format.
4. Sling
- Installed using
pip
and configured on the VM. - With essential paths and endpoints defined in config files to support efficient data ingestion.
5. PyAirbyte
- Installed using
pip
and configured on the VM. - Encountered issues due to limited support for certain data sources like "source-postgres" and "source-mysql".
Key findings
Speed Analysis
Here's how the tools performed in terms of pipeline execution speed:
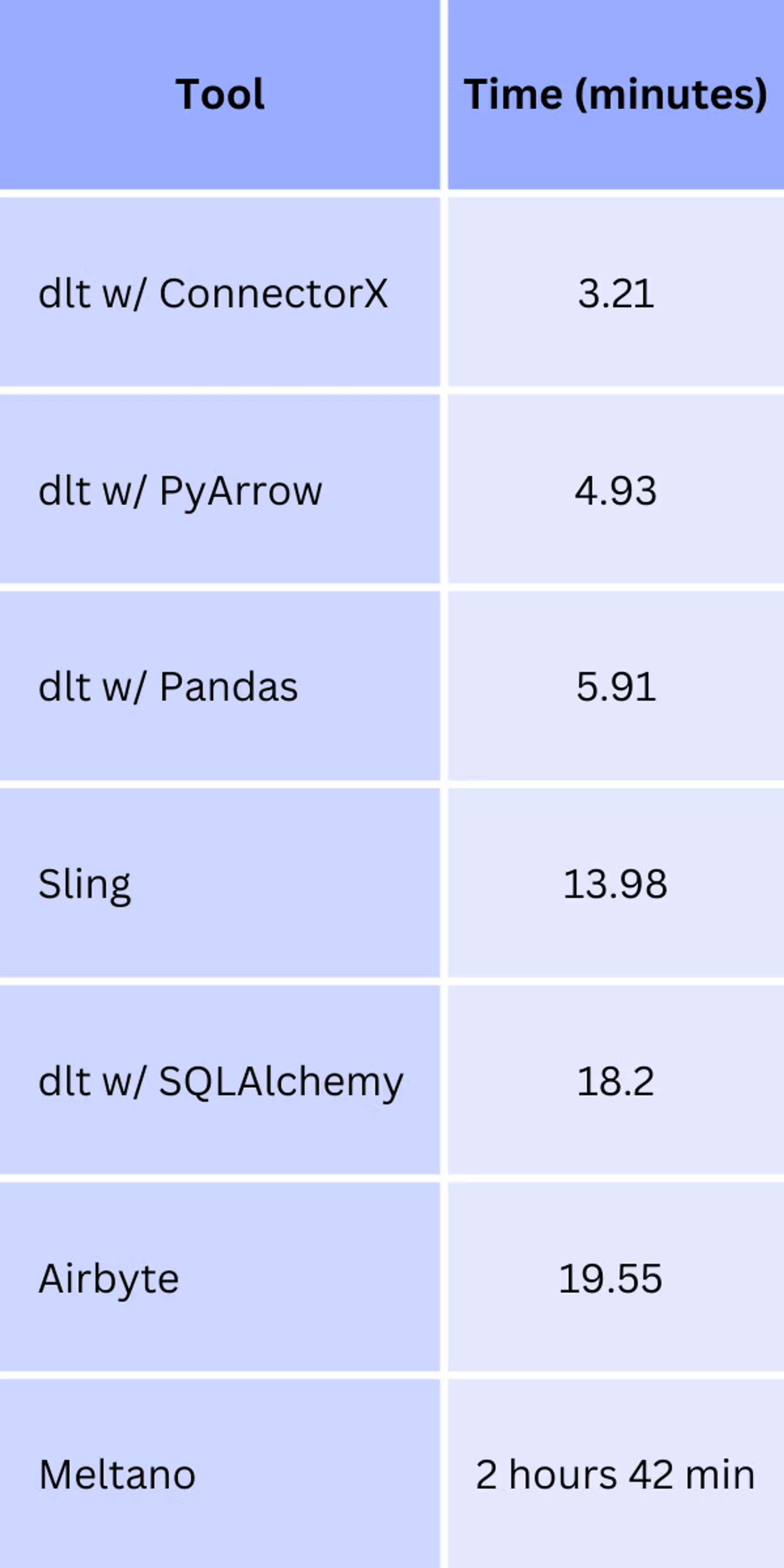
Key Takeaways:
- dlt with ConnectorX and PyArrow is fast, outperforming Sling and Airbyte by 2.8x to 6x, depending on the backend.
- Sling is slightly faster than SQLAlchemy and Airbyte.
- Meltano shows reliable performance but doesn’t match the speed of dlt or other tools in this comparison.
Ease of Use
Here’s how each tool performs in terms of user experience:
1. dlt
- Straightforward setup and user-friendly.
- Compatible with serverless platforms like Google Cloud Functions or Cloud Run, which reduces costs and retains state between runs.
- This makes it an ideal choice for smaller or more extensive data processes.
2. Airbyte
- User-friendly with an interactive web UI, though it requires a constantly running VM.
- Operationalisation is more complicated and requires extra knowledge and effort to manage serverless.
3. Meltano
- Relatively easy to set up and run.
- However, data syncing could be faster compared to other tools, making it better suited for larger, less time-sensitive datasets.
4. Sling
- Lightweight and simple to use, with minimal configuration required.
- Its efficiency makes it a good choice for smaller, near-real-time data processes.
5. PyAirbyte
- Installation was straightforward using
pip
. - However, we encountered challenges due to limited documentation and support for certain data sources.
- As a result, we were unable to fully test PyAirbyte in this benchmark.
Performance:
The two approaches (Infer types vs capture types) scale differently when tuned. The backends can be configured as per requirements. Take a look at ConnectorX and SQLAlchemy backends fine tuning here.
To read more about optimizing dlt please refer to our documentation here.
Conclusion
While SQL-to-SQL data replication is a common need, not all tools perform equally. dlt stands out with its superior speed and ease of use, especially when utilizing ConnectorX and PyArrow. Sling offers a lightweight and efficient solution.
Airbyte and Meltano provide user-friendly interfaces and reliable performance but may require more resources or are better suited for less time-sensitive data.
We look forward to revisiting PyAirbyte once source support is expanded and the documentation is improved.