Fabric + dlt, Course and Explorations
- Adrian Brudaru,
Co-Founder & CDO
Rakesh is a technologist with a track record of delivering secure, scalable cloud and data solutions for both startups and enterprise organizations, especially in domains like IoT, supply chain, and retail.
He focuses on tools that solve problems without adding complexity. This time, he used dlt. He used it because it worked and didn’t get in the way. Different stacks, different problems, and the same ingestion layer that kept showing up and holding up.
You don’t always notice the good tools right away.
They don’t demand attention or sell you on best practices. They just do their job, quietly and predictably, until you realize they’re in half a dozen stacks you’ve built.
That was the case for Rakesh Gupta and dlt.
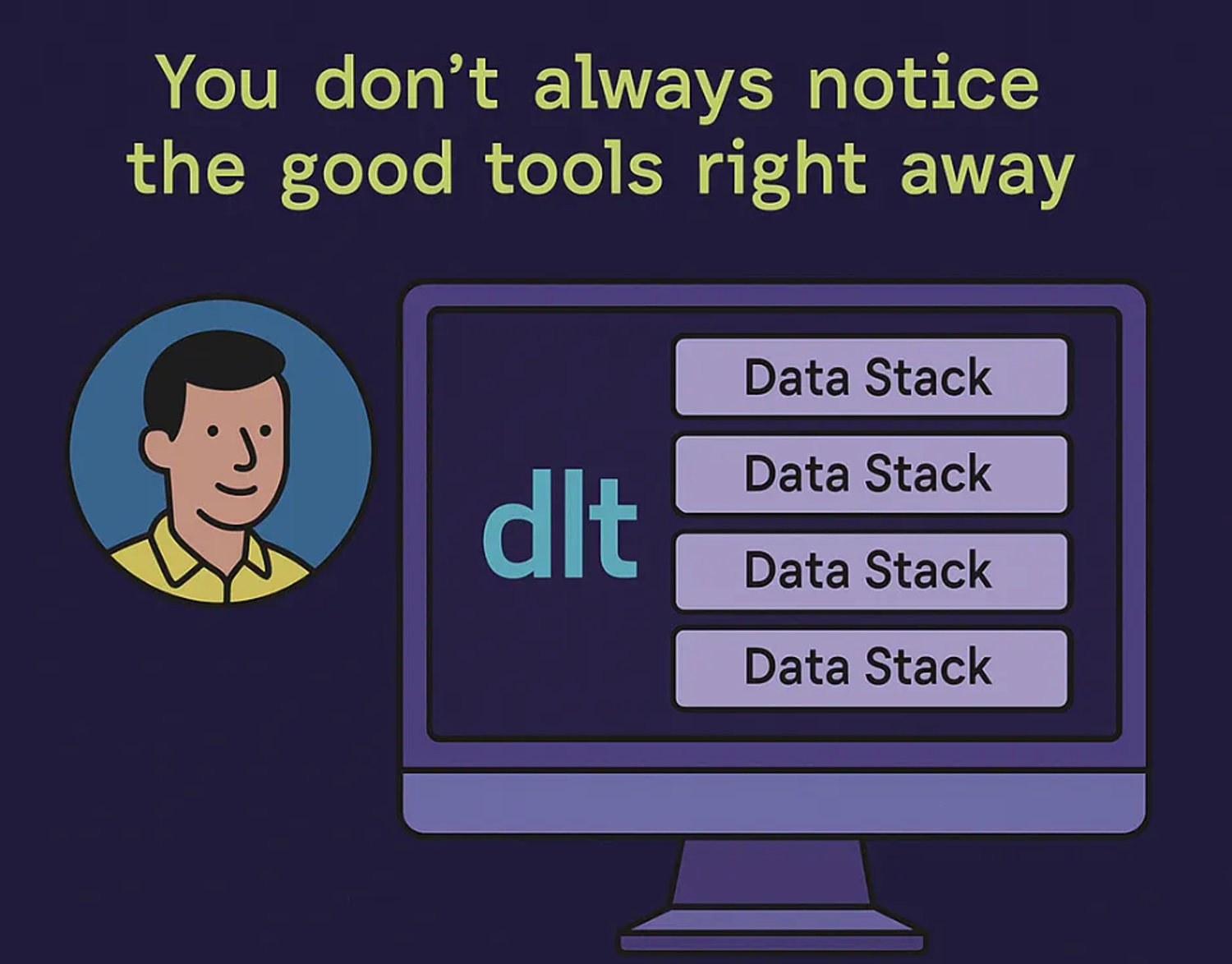
This isn’t a tutorial. It’s a field log: what he built, what stayed simple, and why dlt kept showing up in projects that ranged from AI pipelines to IoT systems to Microsoft Fabric.
Six projects in, and dlt was still the part he didn’t have to worry about.
Here’s what it looked like.
🏠Act I: Forget PowerPoints - a RAG stack for UK property data
Rakesh’s first experiment kicked off with a casual chat with friends about UK property prices. Instead of pulling up a PowerPoint or some static dashboards, he built a RAG stack.
Here’s what he mashed together:
- dlt for ingesting 10+ years of UK housing data
- DuckDB for querying
- Pinecone for vector search
- AWS Bedrock for LLM inference
- Streamlit for charts
What stood out?
- Context is everything. Raw data is just noise until you shape it with clean, relevant data pipelines.
- Make your data speak business. Structuring data semantically opens the door to useful insights.
- Don’t fly blind with AI. If you're tossing around embeddings and tokenization, it helps to actually understand how they work.
The goal was simple. Let people ask real questions about property prices using natural language, not dropdowns and filters.
Was it too much tech for an evening data chat? Definitely. But dlt helped load a decade of CSVs into DuckDB’s clean, queryable rows.
🏠Act II: How Rakesh used dlt to bring edge analytics to life
Rakesh built a solid edge analytics pipeline for IIoT using dlt, DuckDB, and some well-chosen tools. Just the right pieces for the job.
The goal was simple: make machine data useful without over-engineering the stack.
Here’s what he wired together:
- A dlt pipeline that listens to MQTT topics from an EMQX broker
- Messages are saved into daily CSV files, neatly organized by date
- At the end of the day, DuckDB runs analytics over those files
- Results are uploaded to AWS S3 for downstream access
On top of that, he integrated AWS Bedrock and Streamlit, which lets teams ask plain-English questions like: “What were Machine A’s readings yesterday?”
Even cooler? He’s used up agentic apps where the system reads the machine manual for you and says, “Hey, pressure’s low, here’s what to do.”
This setup doesn’t require a complex pipeline or a fleet of engineers to maintain. It’s lightweight, explainable, and deployable at the edge.
🏗️ Act III: Microsoft Fabric, But without spark
Microsoft Fabric assumes you’ll use Spark. Rakesh didn’t. Instead, he used the built-in Python environment, which runs on a single, well-provisioned node, and assembled an end-to-end pipeline. Here’s how:
- dlt to load breach data from “HaveIBeenPwned” into the Fabric Lakehouse
- DuckDB to query and aggregate the data
- MotherDuck to store the results
- Azure Key Vault to manage credentials
- Streamlit for visualization
- Fabric Pipelines to orchestrate the entire flow
Key takeaways
- You don’t always need Spark. Python with dlt and DuckDB can handle large-scale data just fine.
- Fabric’s native features like lineage, monitoring, and scheduling make orchestration feel seamless.
- The setup is flexible. Spark’s still there if you need it, but not every job calls for the heavy machinery.
Just Python, DuckDB, and a pipeline that runs inside Fabric’s native runtime.
📦 Act IV: File-based incremental ingestion
Incremental ingestion sounds simple. It usually isn’t.
Rakesh needed to track new files landing in a Fabric Lakehouse and append only the unseen ones to MotherDuck.
He used dlt’s filesystem source:
- Scanned file metadata to detect new inputs
- Ingested files sequentially using Python
- Tracked modification dates to skip already-processed data
- Appended new records to MotherDuck without touching what was already there
Everything ran in Fabric’s Python runtime. No extra infra, no custom tracking.
📡 Act V: Event-driven, not event-later
Sometimes files just show up. No API. No webhook. Just a blob drop and a timestamp.
Rakesh used dlt and Microsoft Fabric to build ingestion that reacts instead of waits. Here’s what happened:
- Files dropped into the Lakehouse. Just normal data showing up.
- dlt reacted as soon as they landed. No polling, no cron jobs.
- Fabric's Python environment handled the pipeline. Without orchestration overhead.
- Only new files were ingested. No duplicates, nothing reprocessed.
- Dashboards updated automatically. No one had to intervene.
The data landed. The pipeline ran. That’s it.
🔄 It comes full circle
Every platform claims to simplify data workflows. Microsoft Fabric doubled down on that promise. But when Rakesh Gupta followed their recommended approach with Spark and big compute, he found it wasn’t the right fit and went with something simpler and leaner.
So instead, he put together a setup that was easier to manage and didn’t require spinning up extra infrastructure. He used dlt for ingestion, Fabric’s Python environment to run it, and parameterized pipelines to handle both ingestion and transformation and Azure KeyVault to secure things using best practices. The result was a fully native workflow inside Fabric.
Here’s what it did:
- pulled data from the Northwind API
- stored it as JSONL in the Lakehouse, then converted it into Delta tables
- enabled self-service exploration using Fabric’s built-in tools
Everything stayed within Fabric. Simple, contained, and ready for production.
This is the first in a six-part series on working with Fabric’s Python environment. Lightweight, automated, no Spark.
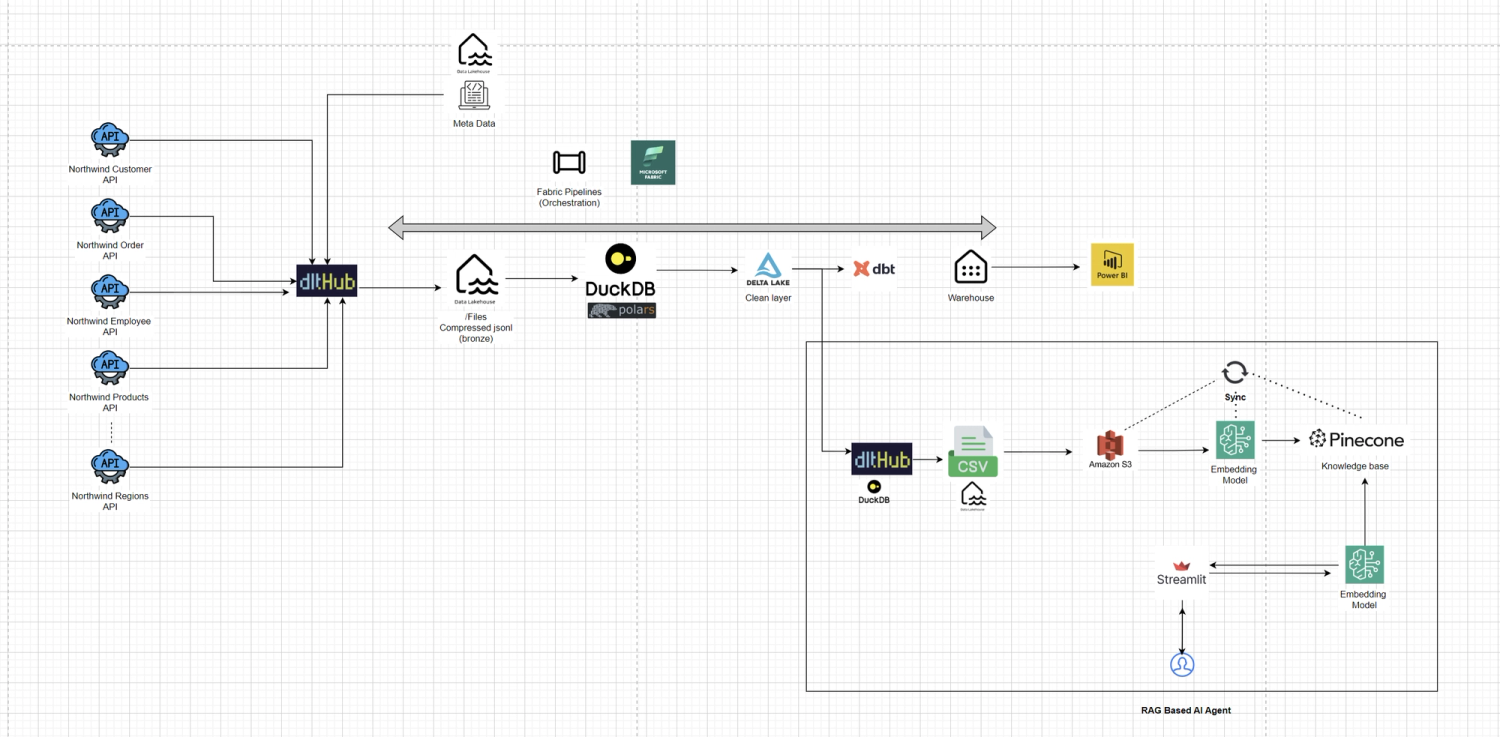
There’s a GitHub repo with all the code.
He’s even hosting a live workshop on it soon.
đź§µ The tool that stayed
Rakesh didn’t plan to keep using dlt. He used it in one stack, then again, and then again.
He tried different tools. Different environments. Different problems. But somehow, the ingestion layer stayed the same, not because it was perfect, but because it was simple, reliable, and good enough not to rethink.
That’s the real test for any tool in data engineering. Not hype. Not benchmarks. Just whether it earns its spot in the next project.
Six stacks later, dlt still had his vote. Not because it did everything right, but because it never got in the way of what he needed to build. And that was the point.